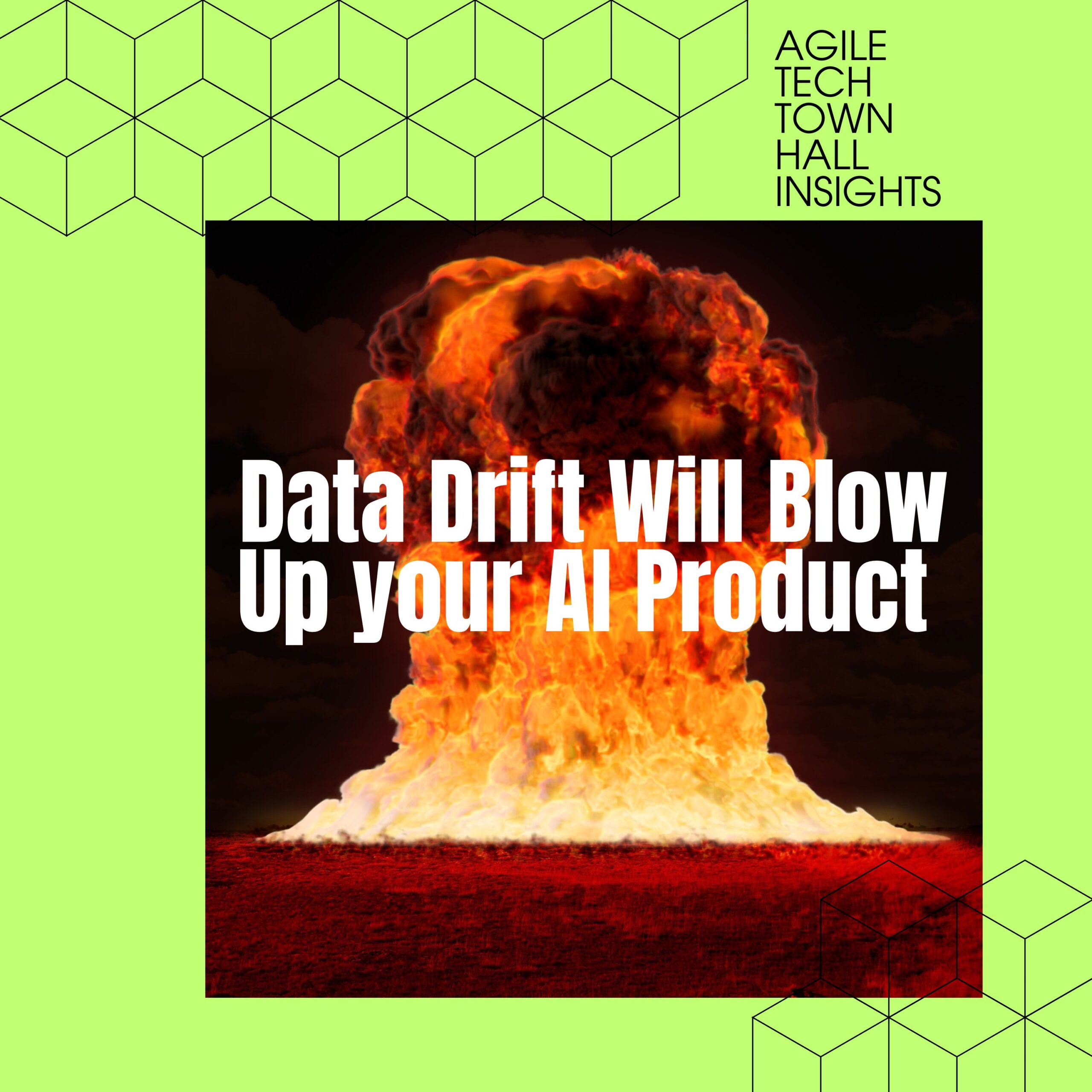
Data Drift Will Blow Up Your AI Product
Have you heard the expression “data drift”?
Last week, Numa Dhamani, a Principal Machine Learning Engineer at KungKuAI, was one of the panelists on our last webinar titled “The Top Reasons Why Your AI Adoption Will Fail.” She explained that AI models are not static entities. They require continuous attention, updates, and retraining to remain effective. Numa emphasizes this point, stating, “Like AI, models need to be maintained. They need to be retrained, they need to be updated. This continuous nature of AI models is both their strength and their Achilles’ heel. While they can adapt and learn from new data, they are also susceptible to what’s known as “data drift.”
**Data Drift: The Silent Challenge**
Data drift refers to the phenomenon where real-world data changes, causing the model’s training data to become less representative. This misalignment can lead to a decay in the model’s performance over time. Dhamani provides a clear example, noting, “What you see with machine learning models is… something called data drift where you’re so far away from the data that it was trained on that you’re going to start seeing a decay in your model.”
A recent study from Stanford University, as reported by Fortune (see link at the bottom), highlighted the real-world implications of this drift. The article titled “Over just a few months, ChatGPT went from correctly answering a simple math problem 98% of the time to just 2%, study finds” reveals the unpredictable effects of changes in AI models. The performance of ChatGPT, a renowned AI chatbot, varied significantly over a few months, underscoring the challenges of data drift.
**The Importance of Quality Data**
At the heart of any AI model lies the data it’s trained on. Dhamani aptly uses the term “garbage in, garbage out” to emphasize the direct correlation between data quality and model performance. She states, “I think the quality of your data directly affects the quality of your model.” Businesses often invest significant resources in research and exploring AI solutions, only to realize they lack sufficient or appropriately labeled data to train an effective model.
**The Need for Transparency and Continuous Monitoring**
One of the significant findings from the Stanford study was ChatGPT’s reduced transparency over time. Initially, the model explained its reasoning for answers, but this behavior diminished over time. Dhamani stresses the importance of transparency, noting the value of having AI models “show their work” so researchers can understand their reasoning processes.
**Resources to Support Your Production Model**
At Agile, We have seen this become a reality for our clients. Our largest team of MLOps engineers is supporting anti-fraud models that support the banking and finance market. This complex model is learning how to hunt down threats throughout a massive and complex financial ecosystem.
If “data drift” is something that will rob us of a solid AI model, what are the top questions we should be asking ourselves before we start these initiatives?
https://fortune.com/2023/07/19/chatgpt-accuracy-stanford-study/